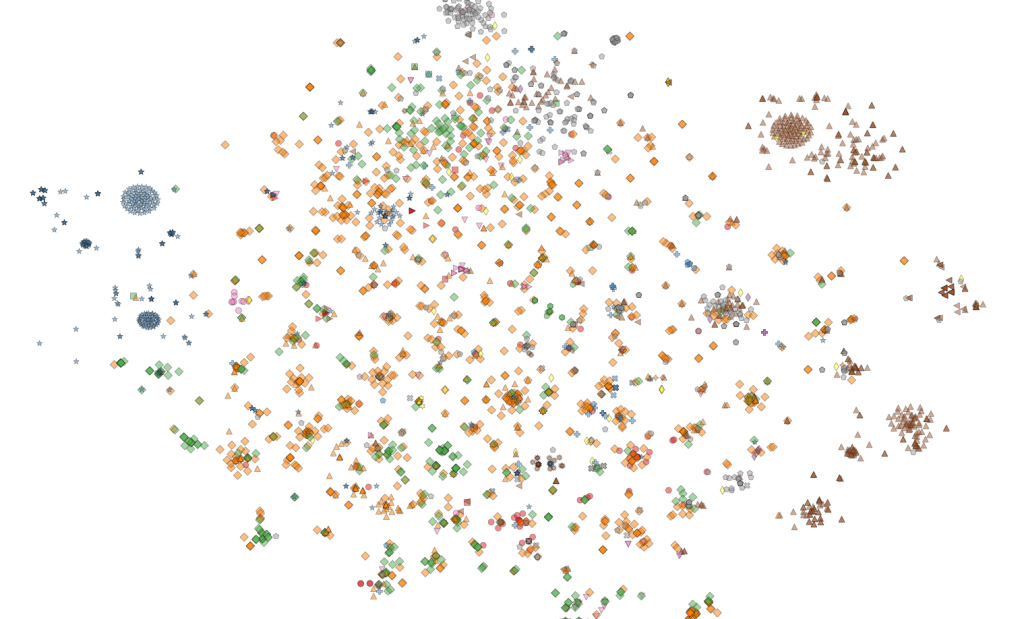
By Darcy Krasne
This project investigates the contours of the CBA’s Fine Art collection as it has been cataloged by successive librarians and is currently represented on the CBA’s website. In particular, it asks whether the object types assigned to individual objects offer a meaningful categorization of the collection; and taking each object’s description and subject keywords in the collection database as a textual proxy for that object, it uses TF-IDF (term frequency–inverse document frequency) to compare the objects based on their “similarity” to each other. The result is a data physicalization consisting of three main parts:
- A quantification of the objects in each object type category, showing where description or subject data is missing from the database;
- networked linkages between object types, showing how closely each is linked to the others through shared subject keywords; and
- visualizations that explore the collected descriptions and subjects of each object type in greater depth and show how well that object type fits the objects assigned to it.
Links: Map of Artist Book Collections in the U.S. | Darcy’s Physicalization Project
Curious about any of the terms used on this page?
Check out our site index which includes basic definitions of some of the concepts we reference.
Suggested Citation:
Darcy Krasne, Bookization: The CBA’s Fine Art Collection Data as Book Art, Books as Art, Art as Data. Pratt Institute: 2024. https://studentwork.prattsi.org/bookarts/bookization-the-cbas-fine-art-collection-data-as-book-art
Proudly powered by WordPress